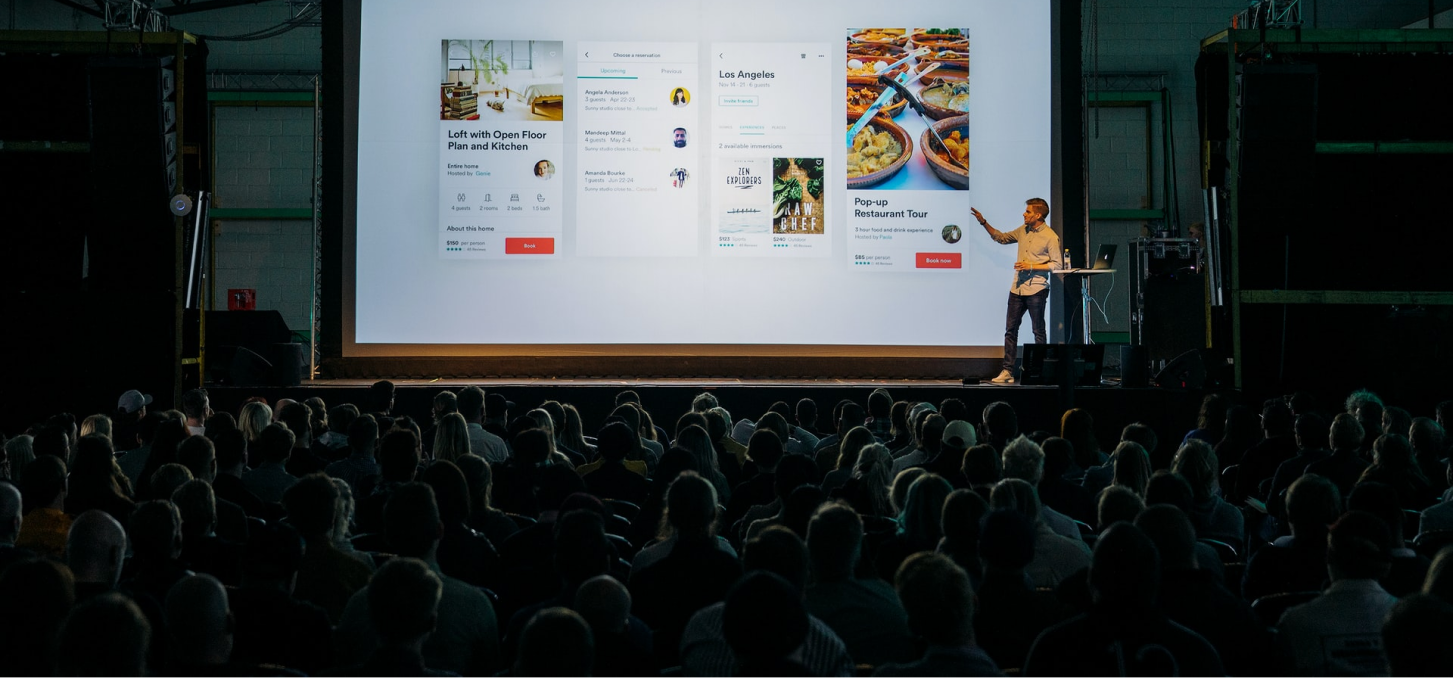
AMLD Africa 2022
Passed Event
Join us for the second edition of AMLD Africa!
3 days of talks, tutorials & workshops, on Machine Learning and Artificial Intelligence with top speakers from industry and academia.
Advances of ML Approaches, Challenges and Opportunities
AI & Agriculture
AI & Environment
AI & Healthcare
AI & NLP
Ines Bahej
activities during the event
Opening Ceremony
Opening/Closing
Hicham El Habti
Hicham El Habti is the President of Mohammed VI Polytechnic University. With a strong background in both corporate and education management, he plays a key role in fostering organizational development, leading the formulation and implementation of the university’s strategic vision, and driving transformation initiatives. His leadership focuses on promoting institutional reforms, enhancing operational efficiency, and establishing impactful collaborations at both local and international levels.
Hicham El Habti has extensive expertise in Applied Mathematics and Economics. He also holds an engineering degree in Economics, Management, and Finance from l’École Normale des Ponts et Chaussées in Paris.
activities during the event
Opening Ceremony
Opening/Closing
Guillaume Scheurer
Guillaume Scheurer earned his Law degree from the University of Neuchâtel and pursued postgraduate studies in International Security Policy at the Graduate Institute of International Studies in Geneva, Switzerland.
His early career included working at a law practice in Neuchâtel, where he was called to the bar, and serving as a lawyer at the general directorate of a large private insurance company near Zürich from 1990 to 1992.
His prior government service includes:
- Ambassador to Ukraine and Moldova (2015-2019)
- Deputy Head of the Delegation to the Swiss Delegation to the OSCE (2013-2015), including during Switzerland’s Chairmanship of the OSCE in 2014
- Deputy Head of the Swiss Embassy in Washington, D.C. (2009-2013), where he also led the Political and Legal Section
- Deputy Head of the Political Division for Human Security, Peace Promotion, and Human Rights at the Ministry of Foreign Affairs in Berne (2005-2008)
activities during the event
Opening Ceremony
Opening/Closing
Nadia Fassi-Fehri
activities during the event
Opening Ceremony
Opening/Closing
Hassan Chafi
Dr. Hassan Chafi is the Vice President of Research and Advanced Development at Oracle, where he also leads the expansion of Oracle’s R&D team in Morocco.
In his role, Dr. Chafi oversees a range of projects, from exploring early-stage research ideas to identifying opportunities for transforming these ideas into advanced software services integrated into Oracle products. His team has made significant contributions to Oracle’s flagship product, the Oracle Database, including the introduction of the new ISO SQL graph query standards, which have been natively implemented in the database.
Dr. Chafi holds more than 60 technical patents and has an extensive background in computer engineering.
Education:
- PhD in Computer Engineering (2014), Stanford University
- MS in Computer Engineering (2006), Stanford University
- BS in Computer Engineering (2004), University of Maryland, College Park
activities during the event
[Invited Keynote] Connecting the Dots: Graph-ML and Beyond
AI & Healthcare
Graph data models are increasingly being leveraged across different critical enterprise workloads. The graph model easily captures data relationships that most data models fail to capture, along with enabling data aggregation from various heterogeneous data assets. This keynote presents Oracle's take on Graph and ML advances along with our journey through industry macro-trends and micro-trends. We also provide practical use cases and business problems that we are solving in healthcare and cyber-security using graph data and ML models and discuss our vision for future enterprise grade data analysis platforms
Nicholas Kelley
Nicholas Kelley is the Director Data Science & A.I. Innovation in the central Novartis Data & AI organization, and has two main priorities. First, to identify, prioritise, and launch key use-cases for the AI Innovation Lab in line with its mission of delivering reusable AI capabilities for the scientific and medical core of the business. Second, on a broader enterprise perspective, to better define the AI opportunities landscape across the value chain. Here the key focus is on continued improvement of our Novartis data science foundation through innovative partnerships and by leading the data science community and capability building initiatives.
Recent initiatives include seeding and driving AI solutions such as Generative Chemistry as part of the partnership with Microsoft Research, the first cross-Novartis Data Science challenge in collaboration with MIT, and launching the AI for Life Residency program. Nick has a formal background in computational methodology with a Ph.D. in Biophysics from Stanford University, with career experience in computational biology, chem- and bioinformatics, and most recently in the application of A.I. and machine learning in health.
activities during the event
[Invited Keynote] - Reimagining medicine at Novartis with the help of AI
AI & Healthcare
We are witnessing a rapid evolution of the healthcare ecosystem boosted by new technologies to better quantify health and bring data driven insights into clinical and pharmaceutical decision making. We will outline the significant challenges associated with conceiving and brining a new drug to market, and highlight the potential that machine learning offers to augment this process via a growing amount and diversity of health data. In particular, personalized and precision medicine are becoming a must for better care. We will dive into specific examples on how machine learning is tailoring drug development via recommended patient characteristics and subgroups, as well as the challenges of working with health data. And lastly, how fresh talents can take their first steps towards launching a career in this space.
Valeria De Luca
Valeria De Luca is associate director and senior principal data scientist at the Novartis Institutes for Biomedical Research, where she works on multi-modal data-driven approaches to quantify patient’s response to disease and treatment. Prior to joining Novartis in 2017, she was a postdoctoral research fellow at UCSF and ETH Zurich focusing on predictive models for critical care. She holds a PhD in Computer Vision from ETH Zurich on personalized cancer treatments.
activities during the event
[Invited Keynote] - Reimagining medicine at Novartis with the help of AI
AI & Healthcare
We are witnessing a rapid evolution of the healthcare ecosystem boosted by new technologies to better quantify health and bring data driven insights into clinical and pharmaceutical decision making. We will outline the significant challenges associated with conceiving and brining a new drug to market, and highlight the potential that machine learning offers to augment this process via a growing amount and diversity of health data. In particular, personalized and precision medicine are becoming a must for better care. We will dive into specific examples on how machine learning is tailoring drug development via recommended patient characteristics and subgroups, as well as the challenges of working with health data. And lastly, how fresh talents can take their first steps towards launching a career in this space.
Fatima-Zahrae Nakach
Fatima-Zahrae Nakach is a first-year Ph.D. student at the UM6P, in the MSDA Lab. She received her engineering degree in Web Intelligence from the Computer Science and Systems Analysis School (ENSIAS, University Mohammed V, Rabat, Morocco) in 2021. Her doctoral research investigates the use of multi-modal machine learning and ensemble learning for the diagnosis and treatment of breast cancer, her work is conducted under the research project “Machine Learning based Breast Cancer Diagnosis and Treatment”
activities during the event
Late fusion of multi-magnification histopathological breast cancer images using deep learning
AI & Healthcare
Breast Cancer is the most frequently diagnosed malignancy and the leading cause of cancer death among women worldwide, the main strategy to reduce mortality is early detection and treatment. Histopathology biopsy imaging is currently the clinically practiced gold standard for diagnosing breast cancer since it captures the effect of the disease on the tissues with a comprehensive view. Pathologists examine the images at various magnification factors to identify the type of the tumor, because if just one magnification is taken into account, the decision may not be accurate, especially if there are variances in the patient’s score at each magnification level. Thus, machine learning models must process the image at various magnification factors for an accurate classification of breast cancer tumors. This work explores the performance of transfer learning and late fusion to construct a multi-modality ensemble that fuses different magnification-specific deep learning models in order to predict the class of the breast cancer histopathological slides.
Mihaela van der Schaar
Mihaela van der Schaar is the John Humphrey Plummer Professor of Machine Learning, Artificial Intelligence and Medicine at the University of Cambridge and a Fellow at The Alan Turing Institute in London. In addition to leading the van der Schaar Lab, Mihaela is founder and director of the Cambridge Centre for AI in Medicine (CCAIM).
Mihaela was elected IEEE Fellow in 2009. She has received numerous awards, including the Oon Prize on Preventative Medicine from the University of Cambridge (2018), a National Science Foundation CAREER Award (2004), 3 IBM Faculty Awards, the IBM Exploratory Stream Analytics Innovation Award, the Philips Make a Difference Award and several best paper awards, including the IEEE Darlington Award.
Mihaela is personally credited as inventor on 35 USA patents (the majority of which are listed here), many of which are still frequently cited and adopted in standards. She has made over 45 contributions to international standards for which she received 3 ISO Awards. In 2019, a Nesta report determined that Mihaela was the most-cited female AI researcher in the U.K.
activities during the event
[Invited Keynote] Panning for insights in medicine and beyond: New frontiers in machine learning interpretability
AI & Healthcare
In this keynote, I describe an extensive new framework for ML interpretability which enables us to turn black-box machine learning methods into white boxes. This framework allows us to unravel underlying governing equations from data, enabling scientists to make new discoveries. Finally, I will introduce our extensive github for ML interpretability: https://github.com/vanderschaarlab/Interpretability
Olorunfemi Situ-Amoo
activities during the event
Using Big Data to drive Health Outcomes
AI & Healthcare
With the widespread adoption of mobile phones, low-cost computing and internet access, significant progress has been made in the digitisation of healthcare systems in emerging markets. More data is being created every day, and the challenge has now become: how can we make use of this data to drive positive outcomes?
Stephen Tashobya
Stephen is the founder and CEO at Wekebere where he’s building the future of prenatal health using wearable technologies and predictive analytics.
Stephen is a passionate entrepreneur on a mission to improve maternal and prenatal health with technology-enabled solutions that accurately monitor the health of mothers and babies. He Loves working at the intersection of engineering, science and healthcare to develop solutions that make people’s lives better.
He is a Software Engineer by training with 10-year experience in R&D and product development in digital health and med tech.
Stephen’s broad experience, ability to build and lead international teams through the development of wearable and digital health products covering hardware, software, data analytics and clinical validation. has seen him deliver strong commercial success, He has won global and continental Awards including Yenching social innovation award 2018 in Beijing china, Innovate for life 2017, ACIA Young ICT Visionaries award 2016, RUFORUM Top 15 young African Entrepreneurs, Innovate for life by Amref Health care, and Youth spark Innovation Grant.
activities during the event
Leveraging data from engaged users to improve maternal and prenatal health using medical grade wearables
AI & Healthcare
In this talk we will show how our data driven approach helps to shed light on poorly understood links between physiological changes and pregnancy outcomes. We will discuss opportunities arising from crowdsourcing clinical research, by providing consumers with clinical grade tools and data, and analyzing such data at a scale beyond what is possible in regular clinical studies. We will take specific examples from Wekebere's research on maternal health, fetal wellbeing and labor detection.
Yoshua Bengio is recognized worldwide as one of the leading experts in artificial intelligence, best known for his pioneering work in deep learning. His contributions earned him the 2018 A.M. Turing Award, often referred to as *the Nobel Prize of Computing*, alongside Geoffrey Hinton and Yann LeCun.
He is a Full Professor at Université de Montréal and serves as the Founder and Scientific Director of Mila – Quebec AI Institute. He also co-directs the CIFAR *Learning in Machines & Brains* program as a Senior Fellow and acts as the Scientific Director of IVADO.
In 2019, he was honored with the prestigious Killam Prize, and in 2022, he became the computer scientist with the highest h-index in the world. He is a Fellow of both the Royal Society of London and the Royal Society of Canada and has been appointed as an Officer of the Order of Canada.
Concerned about AI’s societal impact and ensuring it benefits all, he played a key role in the development of the Montreal Declaration for the Responsible Development of Artificial Intelligence.
activities during the event
[Invited Keynote] Machine Learning for Scientific Discovery
Advances of ML Approaches, Challenges and Opportunities
Machine learning research is expanding its reach, beyond the traditional realm of the tech industry and into the activities of other scientists, opening the door to truly transformative advances in these disciplines. In this lecture I will focus on two aspects, modeling and experimental design, that are intertwined in the theory-experiment-analysis active learning loop that constitutes a core element of the scientific methodology. Computers will be necessary to go beyond the currently purely manual research loop and take advantage of high-throughput experimental setups and large-scale experimental datasets. I will discuss methods related to active learning, reinforcement learning, generative modeling, Bayesian ML, amortized variational learning and causal discovery. I will discuss the notion of epistemic uncertainty and how to estimate it. I will motivate generative policies that can sample a diverse set of candidate solutions to a problem, be it for proposing new experiments or causal hypotheses. Finally, I will describe current research to help us with these questions based on a new deep learning probabilistic framework called GFlowNets and how we plan to apply these in areas of great societal need like the unmet challenge of antimicrobial resistance or the discovery of new materials to help fight climate change.
Sneha Jha
Sneha Jha is a research fellow at Imperial College London where she does interdisciplinary research between the Dept of Mathematics and the Dept of Surgery and Cancer. She is a member of the iCARE group under the NIHR Imperial BRC and the Translational Data Analytics and Informatics In Healthcare group. Prior to Imperial College, she received a graduate degree in computer science from University of Pennsylvania and worked at the Clinical Language Understanding Research group at Nuance. Her research interests are in machine learning and natural language processing with a focus on solving problems in health care. She is also interested in the overlap of technology with policy, law and ethics.
activities during the event
Antipatterns in machine learning in healthcare
AI & Healthcare
This talk aims to discuss issues and common pitfalls during the lifecycle of research projects in the field of machine learning in healthcare. There is a huge gap in the promise and adoption of ML systems in the clinical domain and this talk aims to point out some of the reasons, problems and solutions surrounding this very critical space.
Yves Gaetan Nana Teukam
Yves is a Pre-Doctoral Researcher at IBM Research affiliated with the Technological University of Eindhoven. He has a background in Bioinformatics and Data Science.
During his master thesis, he worked on synthesis planning using deep learning. Part of this work resulted in a publication in Nature Communication in 2022. Yves, as a contributor to the Generative Toolkit for Scientific Discovery, is currently working on generative models for enzyme design using evolutionary-based algorithms.
activities during the event
Enzeptional: enzyme optimization via a generative language modeling-based evolutionary algorithm
AI & Healthcare
Enzymes present remarkable characteristics to enable more sustainable reactions. Our capacity to design effective enzymes in silico has not yet been brought up to speed for industrial research. By viewing enzyme optimization problem as a language modelling task, we can adapt active sites to perform new reactions.
Theophilus Aidoo
Theophilus Aidoo holds a Master of Science degree in Mathematical Science and Bachelors degree in Computer Engineering. He currently works as a remote Data Scientist with Ishango.ai . He is knowledgeable in deep learning algorithms applications in Agriculture.
activities during the event
Land boundary delineation using u-nets
AI & Environment
Land delineation is a particular example of a segmentation problem. In this project we used U-Nets, a type of CNN, to learn the boundaries of parcels of lands from satellite images. The U-Nets model proved very efficient for the task.
Joana Reuss
I am currently withing my first year of my PhD at the Chair of Remote Sensing Technology at the Technical University of Munich, Germany. My research interests lie in the generalization of AI models within the area of Remote Sensing. I am particularly focusing on Meta Learning approaches.
Furthermore, I completed my bachelor’s degree in Business Mathematics at the University of Mannheim, Germany. After two years as a Consultant in the field of Data Science and Analytics, I went on to the University of Barcelona, Spain, in order to complete my M.Sc. in Data Science in July 2021.
activities during the event
Machine Learning approaches for cross-continent transfer of Remote Sensing models for Africa
AI & Environment
Available Remote Sensing datasets are unequally distributed: The Global North has much more labeled data available compared to the Global South. In the project “PreTrainAppEO” we are investigating possibilities of leveraging training data from data-rich regions for applications in data-sparse ones, using recent transfer- and meta-learning approaches.
Elhousna Faouzi
Elhousna FAOUZI from Morocco, I am a 3rd year Ph.D. student at the Sultan Moulay Slimane University in Morocco. My area of interest is Climatology, spatial sciences, Hydrology modelling and Remote sensing. I have been working and conducting researches in this area for 2 years.
activities during the event
Improved water resource management framework using remote Sensing data and machine learning methods
AI & Environment
The introduction presents the context of water scarcity in Morocco and the problem of our thesis. The methodology shows water resources management using rain gauges and remote sensing data as an input in the model. the typical parameter and variables for the model. and finally results and discussion
Ghita Mezzour
Dr. Ghita Mezzour serves as the Moroccan Minister in Charge of Digital Transition and Administration Reform. Before her appointment, she worked as both an entrepreneur and an academic, focusing on the development of digital solutions with significant socio-economic impact. She holds a PhD from Carnegie Mellon University, as well as a Master’s and a Bachelor’s degree from the Swiss Federal Institute of Technology in Lausanne. Dr. Mezzour has been recognized with multiple international awards for her contributions to the field.
activities during the event
[Invited Keynote]
Advances of ML Approaches, Challenges and Opportunities
Foutse Khomh
Foutse Khomh is a Full Professor of Software Engineering at Polytechnique Montréal, Canada CIFAR AI Chair on Trustworthy Machine Learning Software Systems at Mila – Quebec AI Institute, and FRQ-IVADO Research Chair on Software Quality Assurance for Machine Learning Applications.
He received a Ph.D. in Software Engineering from the University of Montreal in 2011, earning the Award of Excellence. In 2019, he was awarded the CS-Can/Info-Can Outstanding Young Computer Science Researcher Prize, Canada’s highest recognition for an early-career academic in Computing.
His research interests include software maintenance and evolution, machine learning systems engineering, cloud engineering, and dependable and trustworthy ML/AI. His work has received four 10-Year Most Influential Paper Awards and six Best/Distinguished Paper Awards.
He has served on the program committees of several international conferences, including ICSE, FSE, ASE, ICSM(E), SANER, and MSR, and has reviewed for multiple top international journals in Software Engineering and AI, including EMSE, TSC, TPAMI, TSE, and TOSEM.
He has also served on the steering committees of SANER (chair), MSR, PROMISE, ICPC (chair), and ICSME (vice-chair). Additionally, he initiated and co-organized the *Software Engineering for Machine Learning Applications (SEMLA)* symposium and the *RELENG (Release Engineering)* workshop series.
He is on the editorial board of multiple international software engineering journals (e.g., *IEEE Software, EMSE, JSEP*) and is a Senior Member of IEEE.
activities during the event
[Invited Keynote] Building Trustworthy Machine Learning Systems
AI & NLP
Abderrahman Skiredj
– Consultant in AI and Operations Research at OCP Solutions from November 2018 to now
– PhD candidate at UM6P-School of Computer Science in NLP from January 2022 to now
– 2015 – 2018 Ecole des Mines de Nancy: French cross-sector engineering school. Majoring in Applied Mathematics. “Major de promotion”, GPA: 3.8/4
– 2017 – 2018 Ecole Polytechnique de Paris: p French Master’s degree in Probability and Finance.
– 2016 – 2017: Université de Lorraine, France: Master in Mathematics in parallel with Ecole des Mines. GPA: 17/20
– 2013-2015: Classes Préparatoires Moulay Youssef, Rabat Maroc, Two-year selective post-baccalaureate university-level courses. Second year in the MP* class
activities during the event
Can Natural Language Modeling be democratized by moving from the classical MLM pre-training paradigm to the Multi-task pre-training one?
AI & NLP
Up to now, Masked Language Model (MLM) framework has been widely adopted for self-supervised language pre-training using large-scale general corpus. Moreover, due to the complexity of natural language, we are witnessing a race for increasingly massive models and this cannot be sustainable. In contrast, there are works arguing the benefit of diversifying tasks in the pre-training phase and other works focusing on efficient ways to positively share knowledge between tasks. This efficient diversification in tasks would potentially give birth to new pre-training paradigms enabling richer language representation and not requiring as much parameters as the classical MLM pre-training paradigm. In this talk, we propose to review these two sets of works namely multi-task pre-training and effective knowledge sharing between tasks, and show some of their implications in the case of Arabic language and its dialects.
Abdellah El Mekki
Abdellah EL MEKKI is a Ph.D. candidate at Mohammed VI Polytechnic University, Morocco working on machine learning and natural language processing (NLP). Mainly, his work is on NLP for low-resource languages. He has published many works on adapting NLP systems for Arabic dialects with zero labeled data. He is interested in transfer learning for NLP and making AI more accessible
activities during the event
Transfer Learning from Modern Standard Arabic to Dialectal Arabic
AI & NLP
With the widespread adoption of natural language processing (NLP) systems through chatbots, writing assistance, and translation... Under-represented languages are still far from having such achievements. This was due to the fact that large amount of data is needed to build such systems, which is not accessible for all languages. Therefore, the challenge has now become: how can we leverage the new NLP achievements to low-resource languages? Abdellah will discuss how to use machine learning to build NLP Systems for low-resource languages, he will present this for the case of the Arabic language and its varieties. These approaches show to achieve high performance for low-resource languages using Zero-Labeled data.
Bakary Diarrassouba
I create strategies, transform them into concepts, and lead creative teams that bring them to life. At the core of my work is building connections through stories. I am currently working on a diverse range of projects at Google, highlighting some of the most historically untold stories.
activities during the event
Building language models, one story at a time
AI & NLP
One-third of the world's languages are spoken in Africa, but less than 1% of African languages are represented online. This is significant because the language you speak, write or sign shapes your online experience. In a Google Research collaboration in Mali, we worked with griots to build a language model for Bambara, one of West Africa's most widely spoken languages. Griots are historians, storytellers, and the real keepers of the Bambara collective memory, passing their knowledge only through storytelling. By recording griots narrating generational stories, we were able to build a model for understanding and translating Bambara speech. We release the findings for the research community and everyone to benefit.
Lawrence Nderu
Dr. Lawrence Nderu is a Lecturer/Research fellow in the Department of Computing at Jomo Kenyatta University of Agriculture and Technology- KENYA. He is also the Dean at JENGA School of Data Science and AI and an Adjunct at Pan African University Institute for Basic Sciences, Technology, and Innovation (PAUSTI). He has a Ph.D. in Computer Science, specializing in Artificial Intelligence and Fuzzy Logic from the University of Paris 8 Vincennes-Saint-Denis, Paris, France, and a Master’s degree in Software Engineering from the Jomo Kenyatta University of Agriculture and Technology. His current research interests are AI and ML in Health, AI and ML in Agriculture, and Natural Language Processing.
activities during the event
Speech Improvement Framework using LSTM Neural Network and Dynamic Time Warping to improve Kenyatta National Hospital records retrieval
AI & NLP
In healthcare, speech recognition is a dominant application of deep learning algorithms. The current systems are more involved in words that are proposed correctly and available in the dictionary, however, algorithms to detect and correct errors are still a challenge. This study will seek to develop a framework based on Long Short-Term Memory (LSTM) neural network and Dynamic Time Warping (DTW) algorithm which also enhances performance on speech signals. The developed framework will also address the issue of accuracy as well as word error rate which is a measure of the performance of speech recognition systems. LSTM neural network is known to have the ability to counter the vanishing gradient problem experienced by recurrent neural networks (RNN) whereas Dynamic time warping is effective in calculating the resemblance between two-time series. This study will adopt the Cross-Industry Standard Process for Data Mining (CRISP-DM) methodology. The developed algorithm will be tested and validated using health records thus giving better performance and increased accuracy.
Elena Sinel
Elena Sinel is a multi-award-winning social entrepreneur committed to revolutionizing education worldwide.
Teens In AI is her latest initiative, driven by her passion for AI and its potential to create a lasting positive impact. Officially launched at the ITU’s AI for Good Global Summit, its mission is to build pipelines for underrepresented talent, enhancing diversity and inclusion in artificial intelligence.
The initiative provides young people aged 12-18 with early exposure to AI for social good through expert mentoring, talks, workshops in AI/ML, human-centered design and ethics, hackathons, accelerators, company tours, and networking opportunities. The vision is to ensure AI is developed by a diverse group of thinkers and doers, advancing AI for the benefit of humanity.
Elena is a highly sought-after speaker. Her recent achievements include:
- Winner – Women4Technology Industry Leadership Award, Mobile World Summit 2020
- Winner – Diversity Award, Mobile World Summit 2020
- 50 Most Influential Women in UK Technology, Computer Weekly, 2019
- CERPrize Winner, 2019
activities during the event
[Invited Keynote] If it isn’t diverse, it isn’t ethical
Advances of ML Approaches, Challenges and Opportunities
Diversity in AI is essential to ensure that the technology we build is fair and just. We must take a hard look at who creates, designs and implements our products and consider how these profucts might impact our society at large. So what does this mean for the future of AI? If we want artificial intelligence to work well, it needs to reflect the societies it serves. It means using artificial intelligence to help us solve the problems we face as a society, rather than just making our lives easier.
Lucilla Sioli
Ms. Lucilla Sioli is the Director for Artificial Intelligence and Digital Industry within the Directorate-General CONNECT at the European Commission.
She is responsible for coordinating the European digitization of industry strategy and developing policies in the fields of artificial intelligence (AI) and semiconductors. The directorate also supports research, development, and innovation in key digital industrial technologies, including microelectronics, photonics, robotics, and AI.
Lucilla holds a PhD in economics from the University of Southampton (UK) and another from the Catholic University of Milan (Italy). She has been a civil servant with the European Commission since 1997.
activities during the event
[Invited Keynote] Artificial Intelligence in the European Union
Advances of ML Approaches, Challenges and Opportunities
Walid Daou
Passionate about how Machine Learning and AI can have an impact on all businesses, Walid Daou currently runs Advanced Analytics initiatives at OCP.
He started his career in Financial Markets, as a Quantitative Trader in London, in Barclays Capital and in a Hedge Fund, Capula.
He then founded and run an Edtech company in France and Morocco for six years, that became the leader in the market of preparation of competitive exams, in Morocco.
Machine Learning enthusiast, he teaches the “”AI for Executive”” course at EDHEC.
Walid Daou is a Graduate from Ecole Polytechnique and Ecole des Mines de Paris, in Applied Mathematics.
activities during the event
[Invited Keynote] AI for industrial and chemical processes
AI & Agriculture
Eslam Hussein
Eslam Hussein arrived in South Africa from his native Egypt in 2013, in February 2014 he enrolled to study Computer Science at the University of the Western Cape. He has since completed his BSc, Hons and finally graduated Magna Cum Laude with an MSc in Computer Science in April 2022.
Today, Eslam works as a Data Scientist at the Inter-University Institute for Data Intensive Astronomy at UWC, where he develops and uses data science projects to teach aspiring data scientists across the continent at hackathons and other events.
activities during the event
Feature Reduction on FT-NIR Spectroscopy to detect Bruised Apples
AI & Agriculture
Spectroscopy data are useful for modelling biological systems. However, using a wide spectrum of wavelengths is not practical in a production setting. Variable selection methods are one efficient way to obtain an optimal model and were the aim of this work. Near-infrared spectral data in the range of 800 – 2500 nm were used to classify bruise damage. Six machine learning classification algorithms were employed, and two variable selection methods were used to determine the most relevant wavelengths for the problem of distinguishing between bruised and non-bruised apples. The selected wavelengths clustered around 900 nm, 1200nm and 1900 nm. The best results were achieved using linear regression and a support vector machine based on up to 40 wavelengths.
Hamza Bendahou
Hamza Bendahou has an Engineering and Financial background, Hamza graduated from SUPAERO in 2012, and worked for 5 years in the insurance field for Gras Savoye WTW where he was managing an actuary team. Passionate about UAVs, he designed and built long range autonomous Drones that can fly up to 4 hours and have onboard multiple sensors targeted to agricultural work. Hamza is an expert in embedded systems, big data insurance analysis and a certified drone pilot that has trained multiple companies to both drone piloting and precision agriculture in more than 12 African countries.
activities during the event
A mobile application to empower smallholder farmers and build bridges between farming & data
AI & Agriculture
SOWIT is an Agtech startup living at the crossroads of remote sensing, AI and agronomy, which goal is to build products fitted to serve the African smallholder farmers, through intense field iterations and user centric design.
Mathurin Videau
activities during the event
Optimizing crops and application to Kenya and other countries in Africa
AI & Agriculture
We combine (i) Geopy’s geographical management tools (ii) the models of crops and crop varieties in PCSE (iii) the optimization capabilities of Nevergrad (iv) NASA’s climate data We generate maps with, at each location: (i) a recommended crop and a recommended variety. (ii) irrigation schedules. Parameters include year (possibly average over several years) and irrigation possibilities, so that we can output results per decade so that climate change is visible and take into account yearly weather forecasts.
Esther Oduntan
Esther Oduntan is a researcher and academia in Computer Science. She obtained her PhD from Ladoke Akintola University of Technology (LAUTECH), MSc in Machine Intelligence from the African Institute of Mathematical Sciences (AMMI) M.Sc, Computer Science from University of Lagos, UNILAG and BSc in Computer Science form Olabisi Onabanjo University, Nigeria Certificate in Education(Computer Science/Economics). She is a Chief Lecturer in the Department of Computer Science at the Federal Polytechnic, Ilaro Ogun State and an Adjunt in Chrisland University, Nigeria. Her research interest is in machine intelligence comprising machine learning, natural language processing, and computer vision. She is a member of Women in Machine Learning (WiML), Black in AI (BAI), Data Science Nigeria (DSN), Women in Data Science (WiMLDS), African Directory of Science, Web of Science Scholar, Computer Professionals of Nigeria (CPN), Professional member of Nigerian Women in Information Technology (NIWIIT) and the Nigeria Computer Society (NCS). Happily married with children
activities during the event
Machine learning for crop disease detection a case study of cassava crop leaves
AI & Agriculture
Cassava is one of Africa’s most important crops found in tropical regions worldwide. As important as cassava is, concerns are raised towards its diseases. In this paper, the aim is to identify and classify images of cassava leaves into healthy and unhealthy (disease) categories using machine learning. The categories include; four(4) disease categories - Cassava Bacterial Blight (CBB), Cassava Brown Streak Disease (CBSD), Cassava Green Mite (CGM) and Cassava Mosaic Disease (CMD), and one (1) health category – Healthy. At the end of this implementation, we were able to train a model that classifies images of the given cassava leaves into one of the five(5) categories.
Abdel Labbi
Dr. Abdel Labbi is an IBM Distinguished Engineer and Head of Data & AI at IBM Research – Zurich. He leads a global team of researchers and engineers in developing the next-generation Hybrid Cloud Data Fabric, designed to provide secure, efficient, and governed enterprise data for AI.
With over 20 years of experience in research, engineering, consulting, and teaching, Dr. Labbi specializes in large-scale Data & AI platforms and digital transformation. He holds a PhD in Applied Mathematics from the University of Grenoble (France) and an MBA from Henley Business School (UK).
Dr. Labbi has collaborated with several Fortune 500 companies on cutting-edge AI projects and has co-authored more than 80 research papers and two books on various aspects of Data and AI theory and applications.
activities during the event
[Invited Keynote] From Big Data to Better Data for Trustworthy AI
Advances of ML Approaches, Challenges and Opportunities
Jörg Schad
Jörg Schad is the CTO at ArangoDB. In a previous life, he has worked on or built machine learning pipelines in healthcare, distributed systems at Mesosphere, and in-memory databases.
He received his Ph.D. for research around distributed databases and data analytics.
He’s a frequent speaker at meetups, international conferences, and lecture halls.
activities during the event
[Invited Keynote] Graph ML - The Next Level of Machine Learning
Advances of ML Approaches, Challenges and Opportunities
This talk focuses on why Graphs have become one of the biggest trends in Machine Learning. Graph Machine Learning based on Graph Analytic Algorithms is driving significant improvements in Fraud/Anomaly Detection, Ranking (Page Rank), Recommendation Engines (collaborative filtering), text summarization, and other NLP tasks. We will cover Graph Analytic Algorithms, their applications, and the more novel–but equally exciting–field of Graph Machine Learning, including topics such as Graph Embeddings, Graph Neural Networks, and applications of Graph Machine Learning. At the end of this session, you will better understand Graph Machine Learning techniques, when to use them, and when to consider other methods
Damien Hilloulin
Damien Hilloulin is a Principal Member of Technical Staff at Oracle Labs Zurich, where he focuses his work on Graph Machine Learning and Recommender Systems projects.
activities during the event
Learning on Graphs with Explainable Graph Neural Networks
Advances of ML Approaches, Challenges and Opportunities
Graph ML provides powerful techniques to address the most difficult ML problems in which the data is connected and can form complex patterns. In this talk we will present Oracle's take on Graph ML advances, and how the different techniques can be applied in several domains.
Mario Viviani
Mario Viviani is Manager of Developer Relations for Omniverse at NVIDIA, based in London, UK. His team focuses on helping developers and partners get familiar and onboard on NVIDIA Omniverse. Passionate technologist and hands on-developer, he’s ex-Amazon, where he led the global Apps and Games Tech Evangelism team; previously was co-founder of startups and led his own consulting company in mobile apps development. International speaker with 200+ past talks, he is always projected into the future and into what is the next “big thing”!
activities during the event
Building with NVIDIA Omniverse: Create Tools for the Metaverse
Advances of ML Approaches, Challenges and Opportunities
The Metaverse is the 3D Evolution of the Internet. 3D virtual worlds and workflows, whether in design or simulation, are now an essential component of every industry, and will require a new set of tools: NVIDIA Omniverse is a scalable platform to build and operate Metaverse applications. In this session we will explore how you can align Omniverse to your business model and build the tools for the virtual worlds of today and tomorrow.
João Pedro Martins
João Pedro Martins leads the AI Technical Strategy team for EMEA, part of Microsoft’s Worldwide Success organization, a team with deep technical skills in AI/ML focused on helping our customers bring their AI workloads to life in Azure.
activities during the event
[Invited Keynote] How are organizations really using AI? A tour of use cases.
Advances of ML Approaches, Challenges and Opportunities
New developments in AI/ML are almost daily, with ground-breaking innovations like GPT-3 or Dall-E2 being announced at regular intervals. But how are organizations worldwide really adopting AI/ML to transform or optimize their business? This session will be a tour of some of the many cases Microsoft Azure AI customers are deploying to transform their organizations, from tabular to forecasting, NLP and Computer Vision.
Andreas Kopp
As a Microsoft Solution Architect for AI and Data Science, Andreas Kopp advises enterprise customers on the planning and implementation of AI based business solutions.
He is focused on Azure Machine Learning, Cognitive Services and Applied AI solutions including medical imaging and responsible AI frameworks and tools
activities during the event
Federated Learning with Azure Machine Learning
Advances of ML Approaches, Challenges and Opportunities
In this demo rich session about Federated Learning, we cover the following items: 1. Need to protect privacy and intellectual property in Machine Learning use cases 2. Understanding the Federated Learning Concept 3. Demo: Deploying and running a global Federated Learning setup on Azure ML
Harmke Alkemade
Harmke Alkemade supports customers in various industries with Data Science and AI use cases that leverage the Azure services, in the role of Specialized AI Cloud Solution Architect in a global team. Previously she worked as a Cloud Solution Architect Data & AI in the Dutch subsidiary of Microsoft. Before joining Microsoft, Harmke followed a graduate program in Artificial Intelligence and worked on various data science and programming projects.
activities during the event
Federated Learning with Azure Machine Learning
Advances of ML Approaches, Challenges and Opportunities
In this demo rich session about Federated Learning, we cover the following items: 1. Need to protect privacy and intellectual property in Machine Learning use cases 2. Understanding the Federated Learning Concept 3. Demo: Deploying and running a global Federated Learning setup on Azure ML
Cyrus Vahid
Cyrus Vahid is a Principal Developer Advocate for AI/ML at AWS. He focuses on delivering engaging talks to inspire builders and customers on how they can use AI/ML to shape their products and services, along with the responsibilities of building such services.
activities during the event
Use AutoML to create high-quality models
Advances of ML Approaches, Challenges and Opportunities
Automatic machine learning, known as AutoML, removes the tedious, iterative, and time-consuming work across the machine learning (ML) workflow from data acquisition to model operationalization, so you can spend less time on low level details and more time on using ML to improve business outcomes. In this session you will learn about the AutoML tools that ake care of sourcing and preparing data, engineering features, training and tuning models, deploying models, and ongoing model monitoring and updating.
Ali Hussein
Ali Hussein is an AI researcher at Ronin Institute. He works in low-resource NLP and AutoML/NAS
activities during the event
An Introduction to PyGlove & Neural Architecture Search
Workshop
PyGlove is a recently open-sourced library by Google that allows programmatically changing code at runtime. This "makes meta-programs much easier to write" to quote the PyGlove development team. Which makes it easy and quick building of powerful AutoML/Neural Architecture Search algorithms. We will explore Neural Architecture Search using PyGlove as our library of implementation/execution. Delivered by: Ali Hussein, Daisy Khaabi,Mazen Mobtassem & Omar Abo El Fotoh, Participants will have an understanding of Neural Architecture Search and how to implement a NAS algorithm using PyGlove.
Ibrahim Yusuf
Ibrahim is an AI Research Engineer at InstaDeep. He is passionate about utilizing AI for the betterment of humanity and has expertise in geo-spatial machine learning, computer vision and computational biology. Ibrahim’s portfolio showcases projects ranging from enhancing food security and disaster response to advancing immunology research and development.research and development.
activities during the event
Introduction to Data-Centric AI
Workshop
Data-Centric AI is an approach to building machine learning models by focusing more on data instead of code. In this workshop, we are going to learn how to leverage data-centric AI techniques to build good models. The outcome of this workshop is as follows: - Understand the importance of quality data on model performance - Learn techniques for improving data quality - Learn how to use tools such as DVC and IPython Widgets. The participants should have knowledge of building models using any deep learning framework. Preferably they should also have a basic understanding of computer vision.
Ibrahim Yusuf
Ibrahim is an AI Research Engineer at InstaDeep. He is passionate about utilizing AI for the betterment of humanity and has expertise in geo-spatial machine learning, computer vision and computational biology. Ibrahim’s portfolio showcases projects ranging from enhancing food security and disaster response to advancing immunology research and development.research and development.
activities during the event
Introduction to Data-Centric AI
Workshop
Data-Centric AI is an approach to building machine learning models by focusing more on data instead of code. In this workshop, we are going to learn how to leverage data-centric AI techniques to build good models. The outcome of this workshop is as follows: - Understand the importance of quality data on model performance - Learn techniques for improving data quality - Learn how to use tools such as DVC and IPython Widgets. The participants should have knowledge of building models using any deep learning framework. Preferably they should also have a basic understanding of computer vision.
Ejiro Onose
I am a machine learning researcher and technical writer.
activities during the event
Building an end to end ML pipeline
Workshop
Amal Tarifa
Amal Tarifa from Tunisia, gets her engineering degree from the National School of Computer Sciences (ENSI) university. In the field of computer sciences, Amal’s Ph.D. thesis (2021) is in the area of Natural Language Processing (NLP), combined with cognitive agents. Amal is also an active researcher at ESPRIT-TECH. She has joined the academic staff of ESPRIT since 2018. She is a scientific member of various known journals and conferences. Besides, she is an NVIDIA certified ambassador
activities during the event
Building Transformer-Based Natural Language Processing Applications
Workshop
Applications for natural language processing (NLP) have exploded in the past decade. With the proliferation of AI assistants and organizations infusing their businesses with more interactive human-machine experiences, understanding how NLP techniques can be used to manipulate, analyze, and generate text-based data is essential. Modern techniques can capture the nuance, context, and sophistication of language, just as humans do. And when designed correctly, developers can use these techniques to build powerful NLP applications that provide natural and seamless human-computer interactions within chatbots, AI voice agents, and more. Deep learning models have gained widespread popularity for NLP because of their ability to accurately generalize over a range of contexts and languages. Transformer-based models, such as Bidirectional Encoder Representations from Transformers (BERT), have revolutionized NLP by offering accuracy comparable to human baselines on benchmarks like SQuAD for question-answer, entity recognition, intent recognition, sentiment analysis, and more. In this workshop, you’ll learn how to use Transformer-based natural language processing models for text classification tasks, such as categorizing documents. You’ll also learn how to leverage Transformer-based models for named-entity recognition (NER) tasks and how to analyze various model features, constraints, and characteristics to determine which model is best suited for a particular use case based on metrics, domain specificity, and available resources. Learning Objectives By participating in this workshop, you’ll: Understand how text embeddings have rapidly evolved in NLP tasks such as Word2Vec, recurrent neural network (RNN)-based embeddings, and Transformers See how Transformer architecture features, especially self-attention, are used to create language models without RNNs Use self-supervision to improve the Transformer architecture in BERT, Megatron, and other variants for superior NLP results Leverage pre-trained, modern NLP models to solve multiple tasks such as text classification, NER, and question answering Manage inference challenges and deploy refined models for live applications
Anant Nawalgaria
activities during the event
Basics of Reinforcement Learning for industrial learning
Workshop
We first introduce the important concepts of RL and Contextual Bandits, followed by their applications in industry, including a technical case-study blog where we applied it to boost user engagement for Digitec Galaxus's newsletter. In the second half, you will do a hands-on lab applying these concepts to make a small recommender system using bandits and RL on Google Cloud, and optionally test your knowledge with a quiz on the course's page on Coursera.
Alexander Erfurt
activities during the event
Basics of Reinforcement Learning for industrial learning
Workshop
We first introduce the important concepts of RL and Contextual Bandits, followed by their applications in industry, including a technical case-study blog where we applied it to boost user engagement for Digitec Galaxus's newsletter. In the second half, you will do a hands-on lab applying these concepts to make a small recommender system using bandits and RL on Google Cloud, and optionally test your knowledge with a quiz on the course's page on Coursera.
Aminat Amusa
Aminat is a Data Scientist at Sidmach Technologies. building cross-functional and scalable data solutions. With background experience in software development, she helps customers choose and implement data solutions tailored to their business needs. She is also the technical lead for the data science team. Aminat is passionate about blockchain and tech communities. She is a leader/organizer at Datafest Africa.
activities during the event
A guide on Blockchain Data Analysis and Model deployment
Workshop
This workshop will go in-depth into how to get blockchain data from apis, visualize and analyze the sample data, train a machine learning model on sample data, and deploy the trained and tested model. After this session, participants will have learned how to get blockchain data from explorers(etherscan, solscan), NFT marketplace(opensea and looksrare) and exchanges(finance), data visualization, and analysis tips and how to train sample blockchain data and deploy the trained and tested model.
Colleen M. Farrelly
Colleen M. Farrelly is a lead data scientist whose industry work spans TDA, NLP, time series, and GIS applications. She’s passionate about data science for social good. She and Dr. Gaba are the authors of The Shape of Data (No Starch Press, 2023).
activities during the event
Time Series Applications. Participants will come away with some theoretical knowledge of time series data and techniques to model time series data, as well as knowledge of how to implement these techniques in Python. It is hoped that the Hackathon part will equip participants with the skills to solve real-world problems in their home countries that involve time series data--tracking climate change to predict future impacts, understanding market and financial instability from events such as the COVID pandemic, testing healthcare interventions over time on reduction of mortality, predicting future food prices in local markets... These can be the start of research papers, government grants, or businesses, and we're happy to help participants build out ideas after the workshop or connect them with those who can help build out the ideas in their home countries.
Workshop
The first half of the workshop will focus on common time series algorithms from a theoretical and applied perspective, including ARIMA/singular spectrum analysis/recurrent neural networks for prediction, topological/geometric approaches for change-point detection, and longitudinal statistical models for statistical testing of factors influencing time series values. The applications will involve Python code and datasets such as the Moroccan stock market, food prices in Burkina Faso, health indicators in Gabon, and climate change in Sudan, among others. Jupyter notebooks and datasets will be provided ahead of time for students to follow along during this half of the workshop, and we'll be making the materials from the talk available to anyone who isn't able to attend but would like to learn. The second half of the workshop will involve a Hackathon using either the Humanitarian Exchange and Zindi open-source datasets from our examples or whatever data participants want to bring. Focus will be on applications that solve problems and could be scaled into papers or businesses. Build teams. Work independently. Come up with a solution in the workshop, or build out a longer-term project!
Yae Gaba
Dr. Yae Gaba is a postdoctoral researcher at Quantum Leap Africa who specializes on geometry and TDA applications. He is putting together a 2023 workshop on TDA to solve practical problems.
activities during the event
Time Series Applications. Participants will come away with some theoretical knowledge of time series data and techniques to model time series data, as well as knowledge of how to implement these techniques in Python. It is hoped that the Hackathon part will equip participants with the skills to solve real-world problems in their home countries that involve time series data--tracking climate change to predict future impacts, understanding market and financial instability from events such as the COVID pandemic, testing healthcare interventions over time on reduction of mortality, predicting future food prices in local markets... These can be the start of research papers, government grants, or businesses, and we're happy to help participants build out ideas after the workshop or connect them with those who can help build out the ideas in their home countries.
Workshop
The first half of the workshop will focus on common time series algorithms from a theoretical and applied perspective, including ARIMA/singular spectrum analysis/recurrent neural networks for prediction, topological/geometric approaches for change-point detection, and longitudinal statistical models for statistical testing of factors influencing time series values. The applications will involve Python code and datasets such as the Moroccan stock market, food prices in Burkina Faso, health indicators in Gabon, and climate change in Sudan, among others. Jupyter notebooks and datasets will be provided ahead of time for students to follow along during this half of the workshop, and we'll be making the materials from the talk available to anyone who isn't able to attend but would like to learn. The second half of the workshop will involve a Hackathon using either the Humanitarian Exchange and Zindi open-source datasets from our examples or whatever data participants want to bring. Focus will be on applications that solve problems and could be scaled into papers or businesses. Build teams. Work independently. Come up with a solution in the workshop, or build out a longer-term project!
Rockefeller
Rockefeller is a PhD student at University of Stellenbosch and sought-after AI evangelist. His research focuses on deep learning applications and dynamic systems.
activities during the event
Time Series Applications. Participants will come away with some theoretical knowledge of time series data and techniques to model time series data, as well as knowledge of how to implement these techniques in Python. It is hoped that the Hackathon part will equip participants with the skills to solve real-world problems in their home countries that involve time series data--tracking climate change to predict future impacts, understanding market and financial instability from events such as the COVID pandemic, testing healthcare interventions over time on reduction of mortality, predicting future food prices in local markets... These can be the start of research papers, government grants, or businesses, and we're happy to help participants build out ideas after the workshop or connect them with those who can help build out the ideas in their home countries.
Workshop
The first half of the workshop will focus on common time series algorithms from a theoretical and applied perspective, including ARIMA/singular spectrum analysis/recurrent neural networks for prediction, topological/geometric approaches for change-point detection, and longitudinal statistical models for statistical testing of factors influencing time series values. The applications will involve Python code and datasets such as the Moroccan stock market, food prices in Burkina Faso, health indicators in Gabon, and climate change in Sudan, among others. Jupyter notebooks and datasets will be provided ahead of time for students to follow along during this half of the workshop, and we'll be making the materials from the talk available to anyone who isn't able to attend but would like to learn. The second half of the workshop will involve a Hackathon using either the Humanitarian Exchange and Zindi open-source datasets from our examples or whatever data participants want to bring. Focus will be on applications that solve problems and could be scaled into papers or businesses. Build teams. Work independently. Come up with a solution in the workshop, or build out a longer-term project!
Join the event